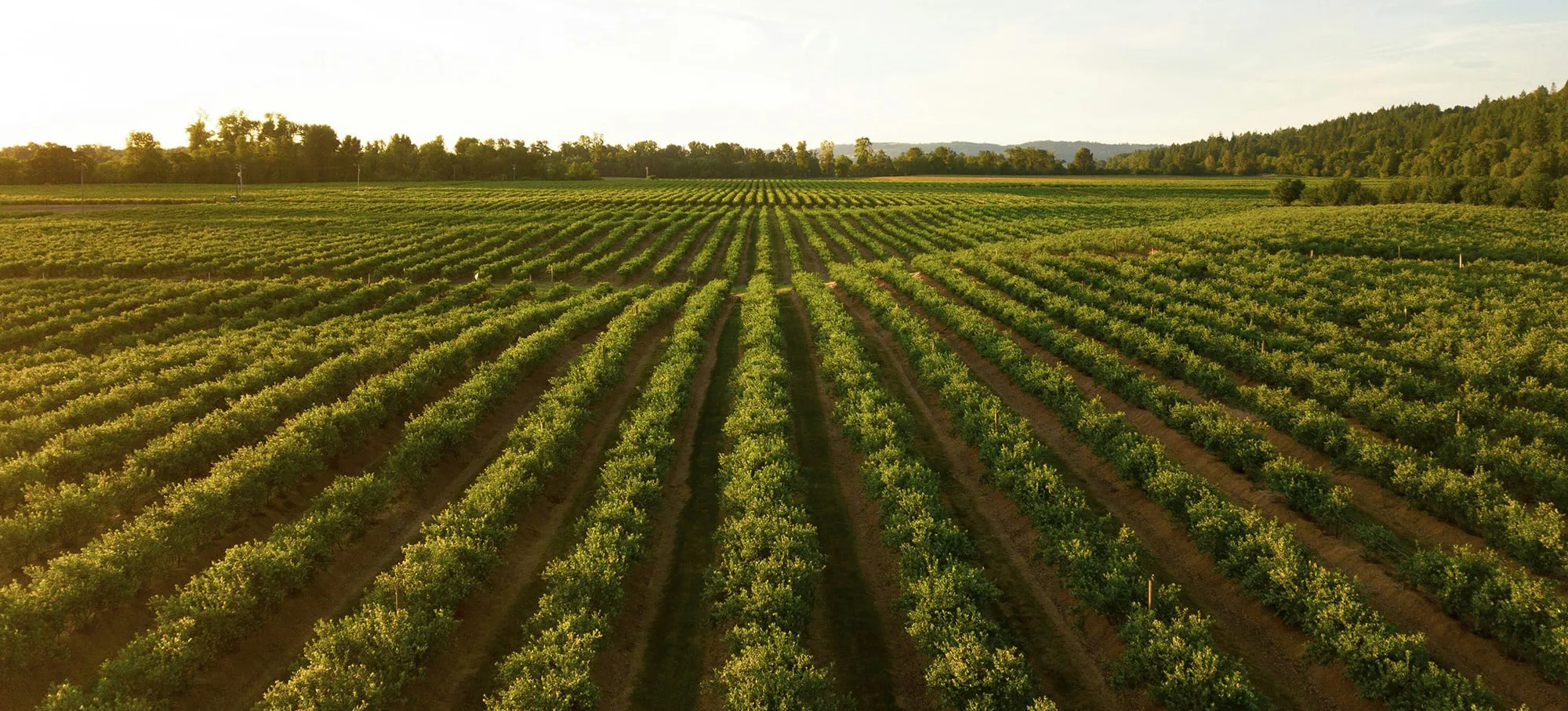
Causal inference for food insecurity analysis
Use Case 3 will explore Causality for food security under Climate Change
Background
In recent years, food insecurity has become a major public concern worldwide. The scale of the global hunger and malnutrition crisis is enormous, with an expected 345.2 million people projected to be food insecure in 2023, more than double the number in 2020. The causes of this crisis are multiple and complex, including poverty, economic crises, climate change, land degradation, conflicts, and inadequate resource access. Climate change has a significant impact on food security, both by reducing crop yields due to heat waves and droughts and by damaging agricultural infrastructure and reducing the amount of arable land available due to extreme weather events, such as floods and storms. Understanding the underlying causes of food insecurity is pivotal for improving the effectiveness of humanitarian actions. Food insecurity levels are currently estimated using a variety of data sources, and the most widely used classification is the Integrated Food Security Phase Classification (IPC), which was developed to achieve consensus on food insecurity levels by following a set of protocols. IPC classes are determined by a panel of domain experts who evaluate the severity of the crisis, its main drivers, and the number of people affected.
Gaps
Estimating food insecurity in different countries has certain shortcomings. Firstly, the process is consensus based, requiring the involvement of multiple stakeholders, making it difficult to reproduce on a large scale. Secondly, it is not automated and does not use unbiased models to exploit the large amount of available data. Thirdly, it provides limited information on the causes of food insecurity and how different factors interact with each other. To address these issues, the use of causal inference and representation methods, combined with big Copernicus data and socioeconomic indicators, can help us better understand this complex phenomenon and improve current policies and actions to reduce food insecurity in vulnerable areas.
Objectives
- Develop a new approach to learn feature representations and understand the target food security indicators, (e.g., Food Consumption Score (FCS) & Reduced Coping Strategies Index (rCSI)), from multiscale and multisource data,
- demonstrate the use of causal Machine Learning to link the impacts of climate and socioeconomic factors on food insecurity,
- enhance food insecurity risk assessment by enabling the interpretation and evaluation of specific interventions’ likelihood and potential consequences.
Scale
The AOIs will be selected in the Horn of Africa, vastly impacted in recent years by complicated relations of concomitant drivers: food prices, production, energy shortage, severe droughts, lack of humanitarian aid, etc.
Technical Partners
The Use Case will be developed by UVEG in close collaboration with World Food Programme.
Co-design users
WFP, which over the years has introduced, developed, and maintained multiple food insecurity indices, such as the FCS. We will make use of data available through WFP’s Hunger Map LIVE.
Links to ThinkingEarth pillars:
- Copernicus foundation models: SSL representations for LULC,
- Earth as a Graph: use learned teleconnections as inputs for food insecurity representation learning,
- Causal models: algorithms for learning causal representations of food insecurity indices.
Interested in collaborating with us?
Contact Prof. Gustau Camps-Valls (gustau.camps@uv.es), or Dr. Miguel-Ángel Fernández-Torres (miguel.a.fernandez@uv.es), Universitat de València, València, Spain.