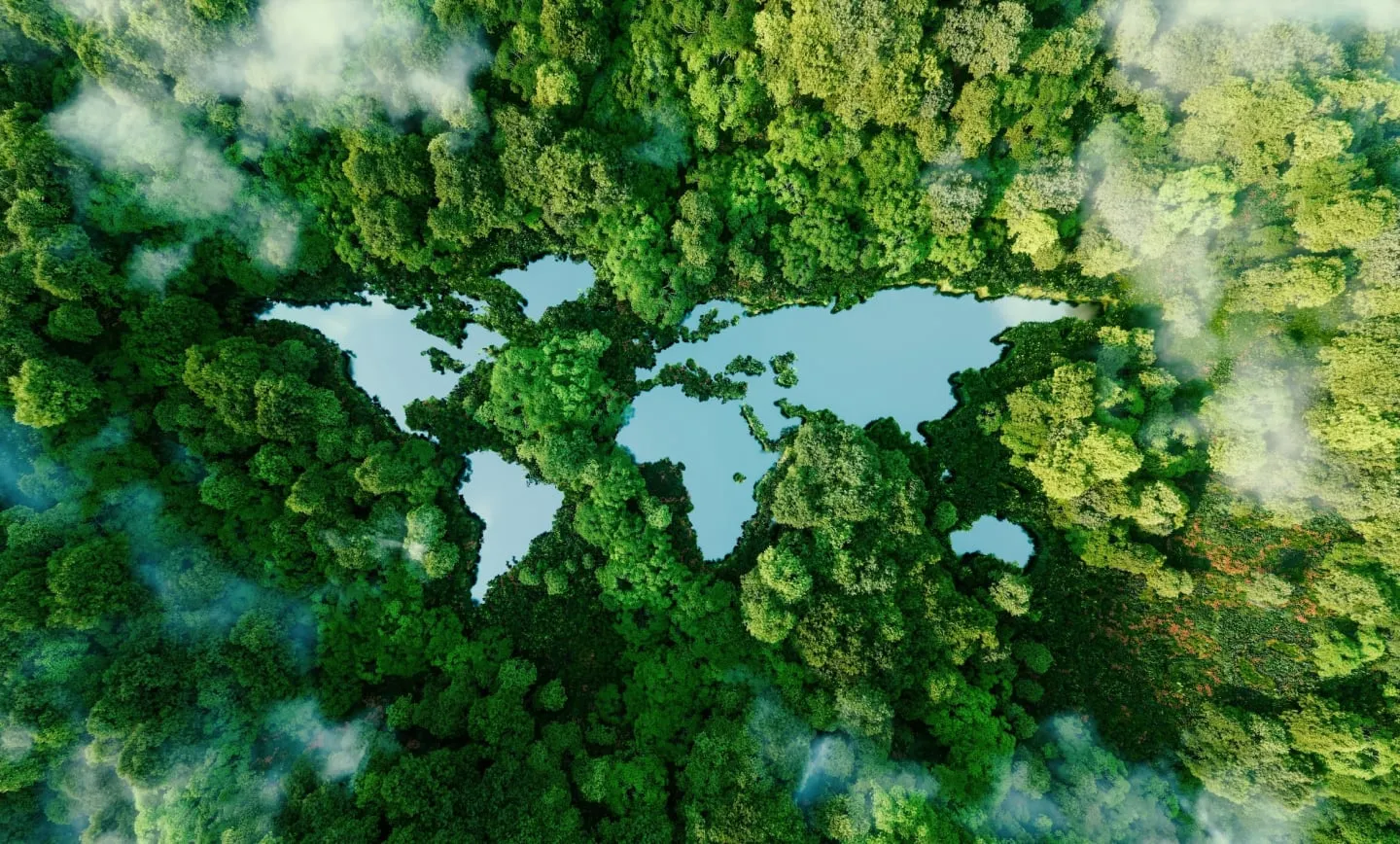
The ThinkingEarth Project
At ThinkingEarth, we view the Earth as a complex unified and interconnected system.
To harness the power of Artificial Intelligence (AI), we use cutting-edge techniques, including deep learning, causality, eXplainable AI, and physics-aware Machine Learning. We leverage the predictive abilities of Self-Supervised Learning and Graph Neural Networks to develop task-agnostic Copernicus Foundation Models and a Graph representation model of the Earth.
We demonstrate the potential of these assets through small-scale downstream Spotlight Applications, as well as large-scale use cases that integrate distributed industrial and user non-EO datasets. These use cases address ambitious problems with high socio-environmental impact and new business growth opportunities, such as accelerating Europe’s clean energy transition and independence from volatile fossil fuels, understanding Earth’s processes by modelling causal Earth system teleconnections, and assessing and modelling the impact of current and future Climate emergency in biodiversity and food security.
Objectives
Research & develop AI methods that treat Earth as a complex unified, interconnected system.
The main objective of ThinkingEarth is to create the first Copernicus Foundation Models based on multimodal Sentinel data and create a data-driven graph representation of the interactions of fundamental earth system variables (ESV) towards modelling the Earth as a Graph. We will demonstrate their value through small-scale spotlight applications and large-scale Use Cases.
Research and develop the first Copernicus Foundation Models
We’ll explore self-supervised learning techniques tailored for Copernicus Sentinel datasets, with a focus on improving contrastive learning and masked image modelling for Sentinel-1, 2, and 3 data compression. Our approach involves developing methods to fuse self-supervised representations from various Sentinel data types, retaining valuable information while considering modality dominance. Additionally, we aim to enhance the generalisation of deep learning models across space and time using self-supervised learning embeddings, meta-learning, and continual learning strategies.
Research and develop a DL representation of the Earth as a graph
Our aim is to construct a foundational Earth model using graph neural networks (GNNs) to depict connections between events across various locations and times. This involves developing innovative spatio-temporal GNN architectures capable of analysing both static and dynamic Earth representations, facilitating the understanding and prediction of climate variability on regional and global scales. By employing self-supervised graph learning techniques, we’ll train these architectures to capture interactions among Earth system variables, contributing to the DestinE initiative through the creation of a prototype Earth graph model integrated with NVIDIA’s Earth-2 engine for visualisation on the Omniverse platform.
Apply causal methods, physics-aware ML, and xAI to solve earth system science problems
We’ll employ a hybrid modelling approach to create AI models that blend physics-based constraints, enhancing consistency. To improve transparency, we’ll utilise causality and explainable AI techniques like feature selection, neuron visualisation, and causal inference. Analysing multimodal remotely sensed data, we’ll uncover cause-and-effect relationships such as teleconnections and memory/lag effects, addressing scientific inquiries such as the impact of different Earth system variables on sub-seasonal climate variability and the predictive value of teleconnections for seasonal climate patterns.
Develop novel, scalable, products utilising Copernicus and non-EO industrial/user data
Using Copernicus Foundation Models and Earth as a Graph model, ThinkingEarth aims to create new value chains in renewable energy, biodiversity management, and food security. We will develop services, such as city scale short-term solar energy forecasting, a smart grid energy management system that combines distributed solar-powered household energy supply predictions and demand data, continuous monitoring of urban biodiversity, forest carbon credit assessments for international financial institutions (IFIs) using above-ground biomass (AGB) estimates and learning causal feature representations of food security indicators. These data-driven causal graphs will help improve seasonal forecasting of food crises and make WFP Anticipatory Action (A-A) more efficient.