Enhancing Land Cover Mapping with ThinkingEarth’s Self-Learning Techniques
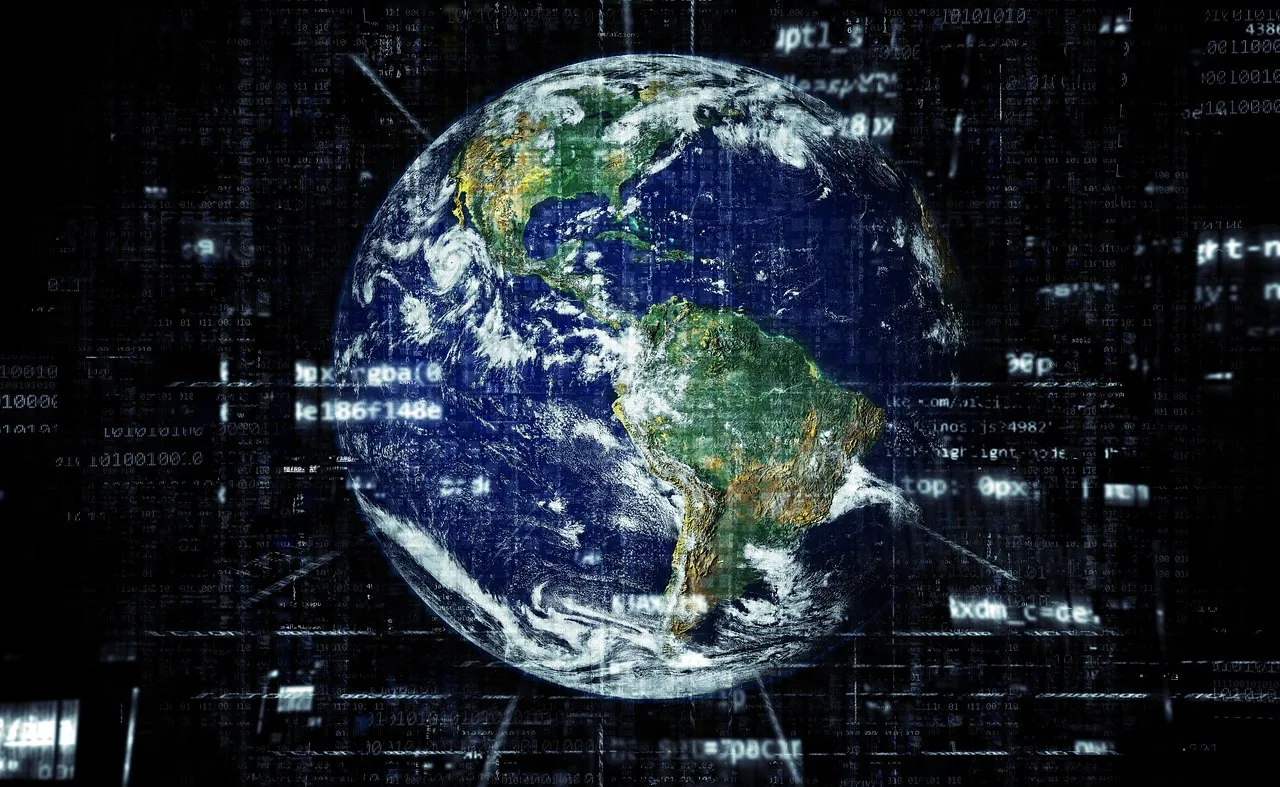
How Self-Supervised Learning Helps with Remote Sensing
Self-supervised learning is a method where the system teaches itself by creating its own labels from unlabelled data. This helps models learn useful information without needing a lot of human input. In remote sensing, SSL helps in these ways:
- Finding Important Features: ThinkingEarth’s SSL technique pull out key details from satellite images, such as colours, shapes, and patterns, that might be missed by traditional methods.
- Less Need for Manual Labels: SSL reduces the need for large, labelled datasets, saving time and money. This makes it easier to map land cover in places where labelled data is hard to get.
- Stronger Models: SSL helps models work well even with data from different types of satellites and locations, making them reliable in various conditions.
Developing the First Copernicus Foundation Models
ThinkingEarth is working on creating the first Copernicus Foundation Models. This involves using self-supervised learning techniques specifically designed for Copernicus Sentinel datasets, including Sentinel-1, Sentinel-2, and Sentinel-3. The focus will be on improving contrastive learning and masked image modelling, which will help compress data effectively without losing important details.
Our approach aims to combine self-supervised data from different Sentinel sources to retain valuable information while balancing the strengths of each data type. We also plan to enhance the ability of deep learning models to perform well across different regions and time periods. This will be achieved by combining self-supervised learning machine learning, among other techniques, ensuring models stay accurate as new data becomes available.
Where This Technology is Useful
ThinkingEarth’s SSL-enhanced mapping can be used in many areas:
- Environmental Tracking: Better detection of deforestation, urban growth, and wetland changes.
- Farming: Improved crop monitoring and management.
- Disaster Response: Quick and accurate mapping of areas affected by floods, fires, and storms.
Conclusion
ThinkingEarth’s self-supervised learning methods are changing how we map land cover. By using unlabelled data properly, mapping can be made faster, cheaper, and more accurate. This technology helps us better understand and protect our environment, now and in the future.
Share
Read next
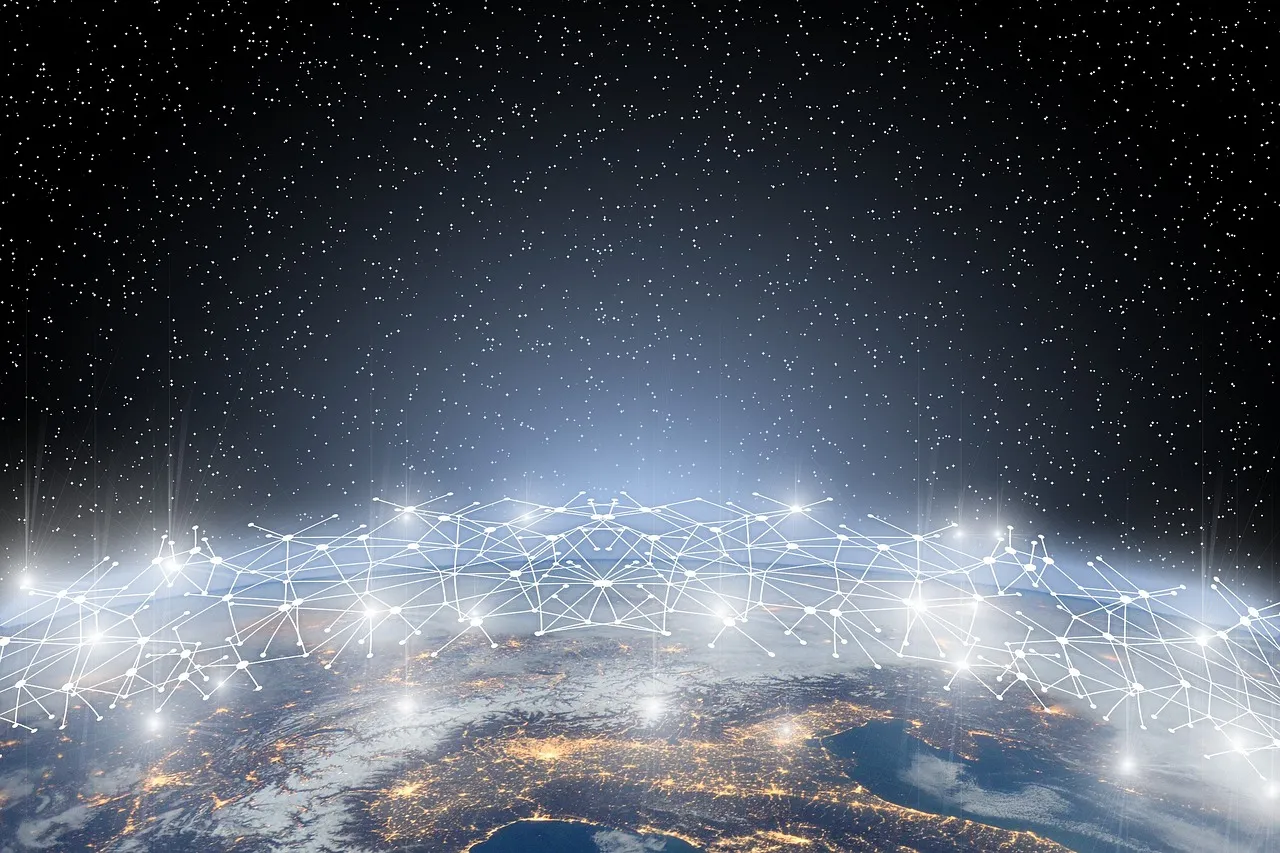
Graph Neural Networks and ThinkingEarth
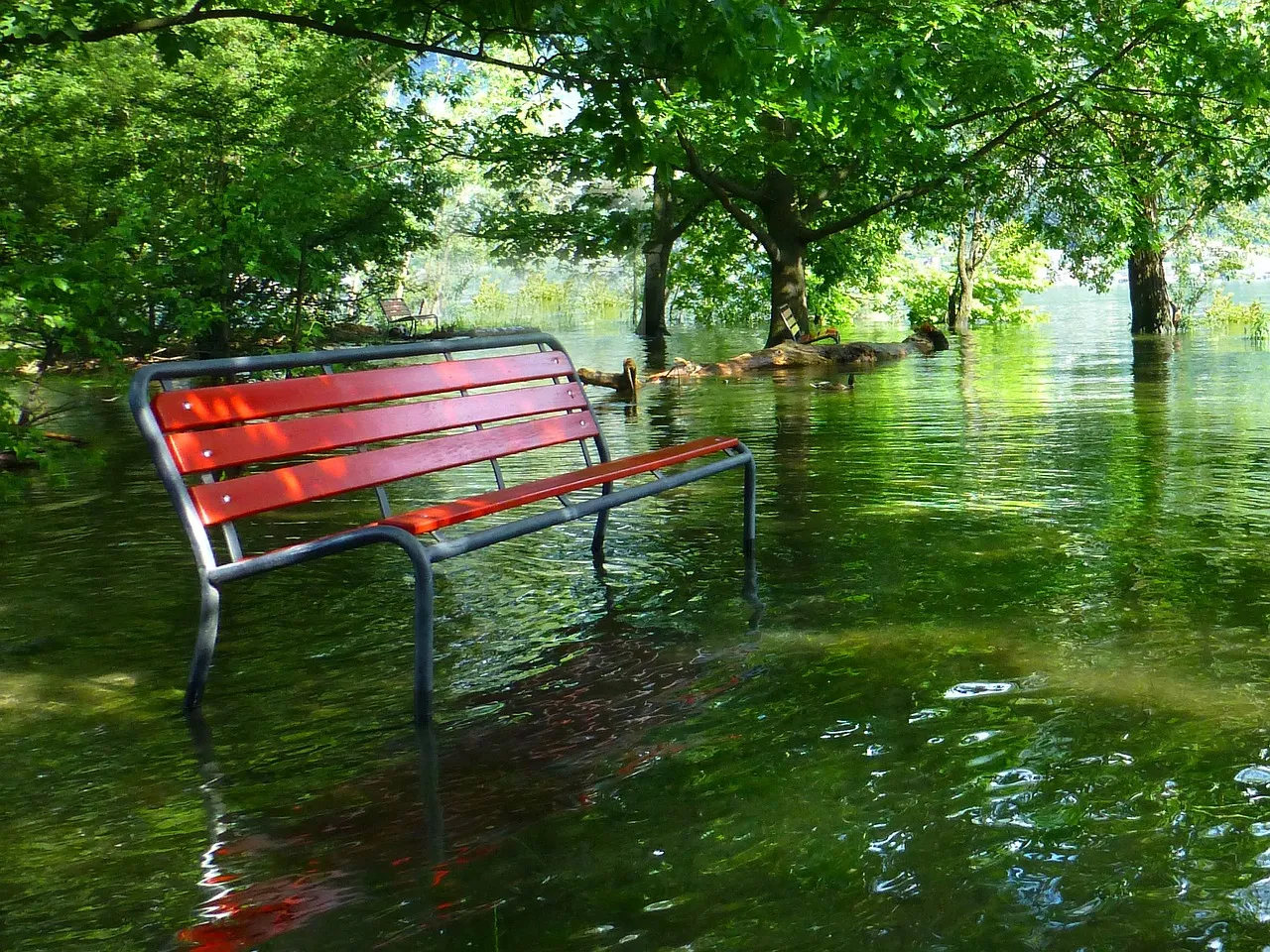
ThinkingEarth and Flood Mapping: Enhancing the Future of Disaster Response
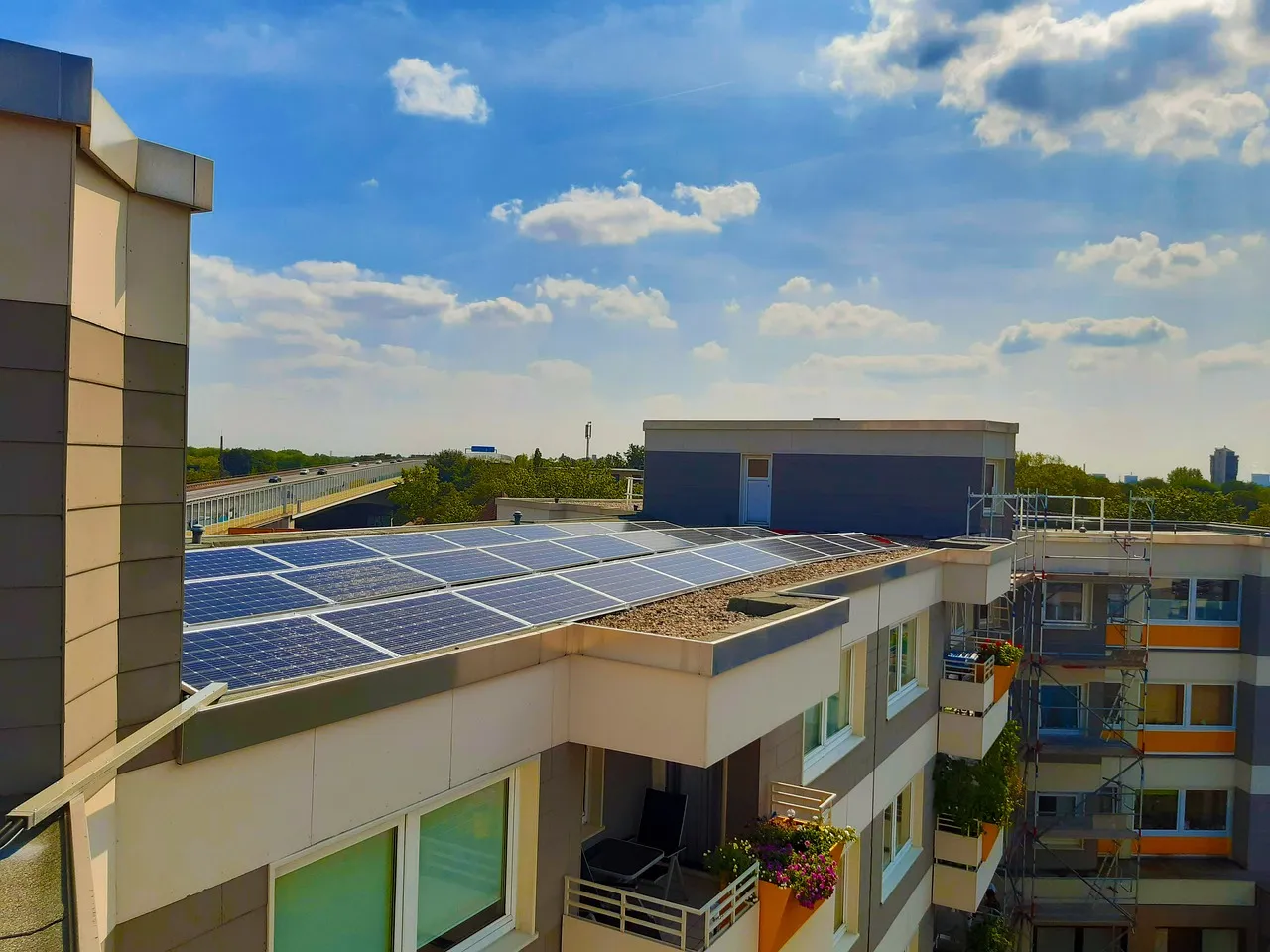