Graph Neural Networks and ThinkingEarth
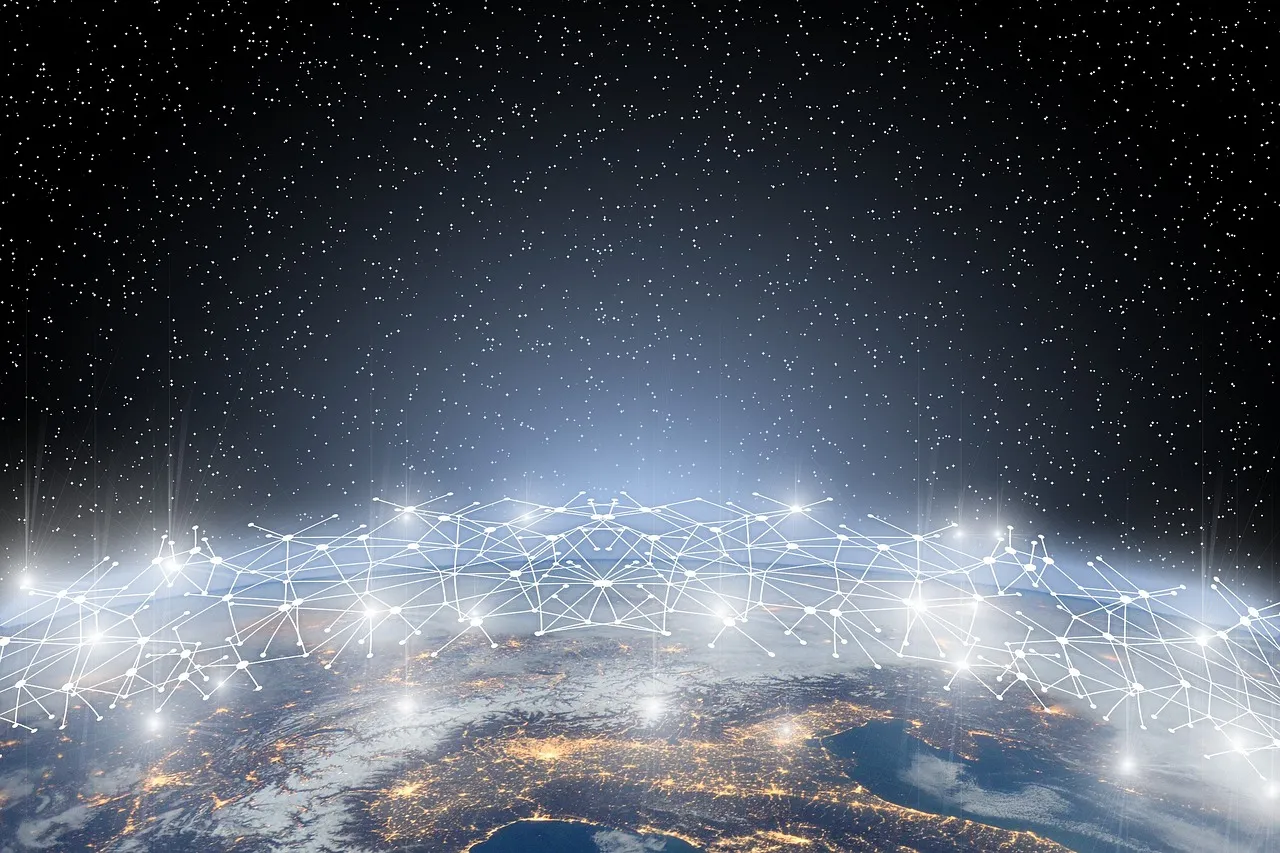
Graph Neural Networks (GNNs) are advanced machine learning models designed to analyse graph data by modelling the relationships between connected nodes. These networks can aid in recognising patterns across various ecosystems, predicting links between them, and analysing entire graphs.
Building the Earth as a Graph
ThinkingEarth’s goal is to represent the Earth as an interconnected system.
This involves:
- Prototype Earth Graph Model: Building a foundational graph-based Earth model with GNNs.
- Visualisation Integration: Partnering with NVIDIA to visualise the Earth graph model using the Earth-2 engine on the Omniverse platform.
This graph framework allows us to capture the relationships and dependencies between different parts of the Earth’s systems, forming the basis for advanced analysis and prediction.
Innovative Spatio-Temporal GNN Architectures
To deal with the complexity of Earth’s dynamic systems, ThinkingEarth develops specialised spatio-temporal GNN models that:
- Analyse static structures, such as geographical features.
- Model dynamic processes, like changing weather patterns or seasonal shifts.
These architectures enable the model to understand both stable and evolving aspects of Earth’s systems, creating a comprehensive and adaptable representation.
Self-Supervised Graph Learning
Self-supervised learning is crucial for training GNNs effectively, especially when dealing with vast amounts of Earth data. This approach allows the model to:
- See patterns from self-supervised learning techniques tailored for Copernicus Sentinel datasets, with a focus on improving contrastive learning and masked image modelling for Sentinel-1, 2, and 3 data compression.
- Capture relationships between Earth system variables, such as the effect of ocean temperatures on atmospheric conditions.
This makes the model robust and capable of pattern recognition across diverse scenarios.
The Earth graph model has numerous applications, including:
- Climate Prediction: Understanding and forecasting climate changes at local and global scales.
- Disaster Management: Identifying early signals for natural disasters like floods or hurricanes.
- Sustainability Efforts: Helping plan for urban development, agriculture, and resource management.
By combining cutting-edge GNN technology with Earth system science, ThinkingEarth aims to revolutionise how we address environmental challenges.
ThinkingEarth’s use of GNNs to model the Earth represents a major advancement in AI-driven environmental science. These innovations enable better understanding, prediction, and management of our planet’s dynamic systems. Through contributions to initiatives like DestinE, ThinkingEarth demonstrates the potential of AI to create actionable insights for a more sustainable future.
Share
Read next
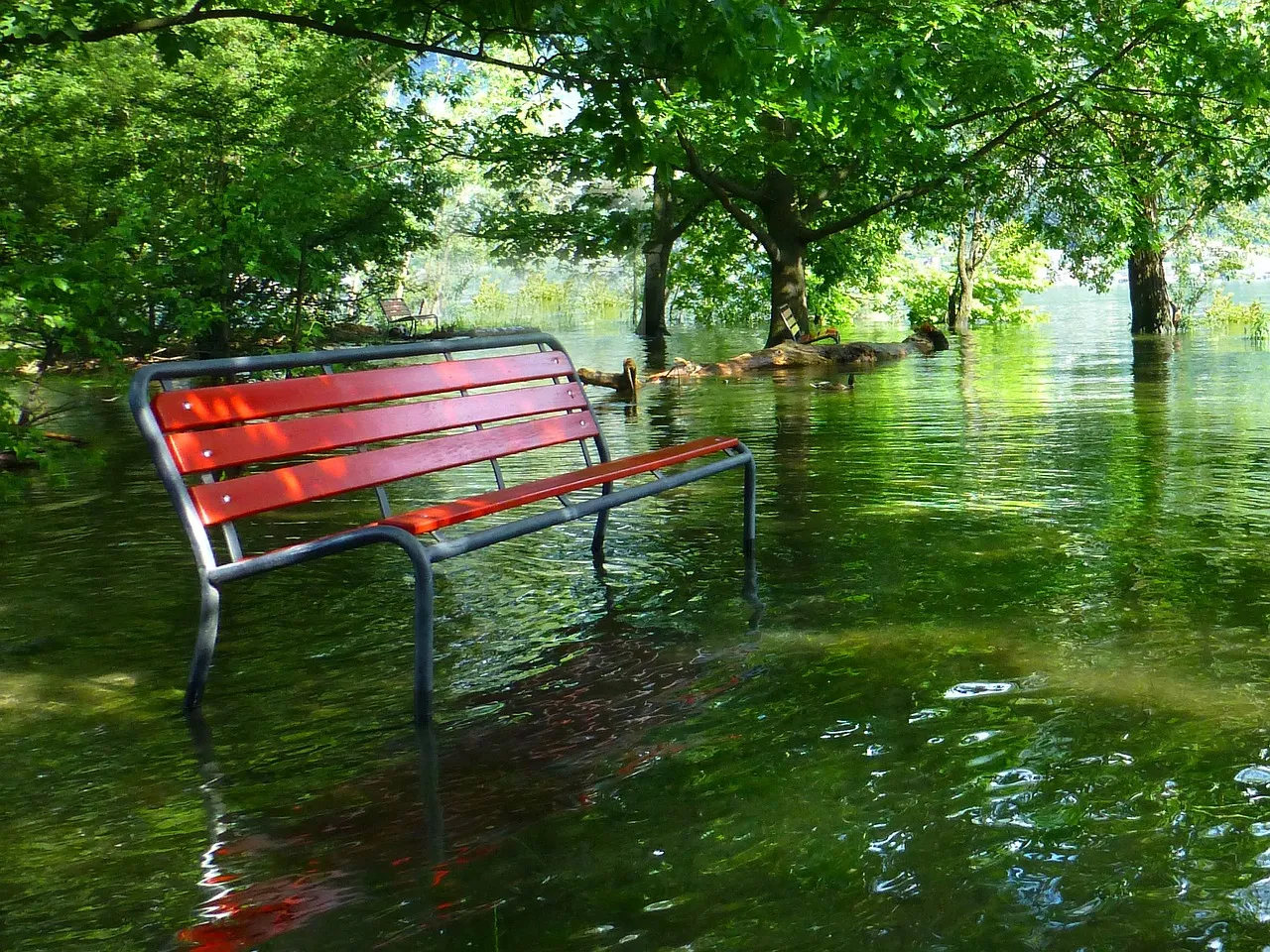
ThinkingEarth and Flood Mapping: Enhancing the Future of Disaster Response
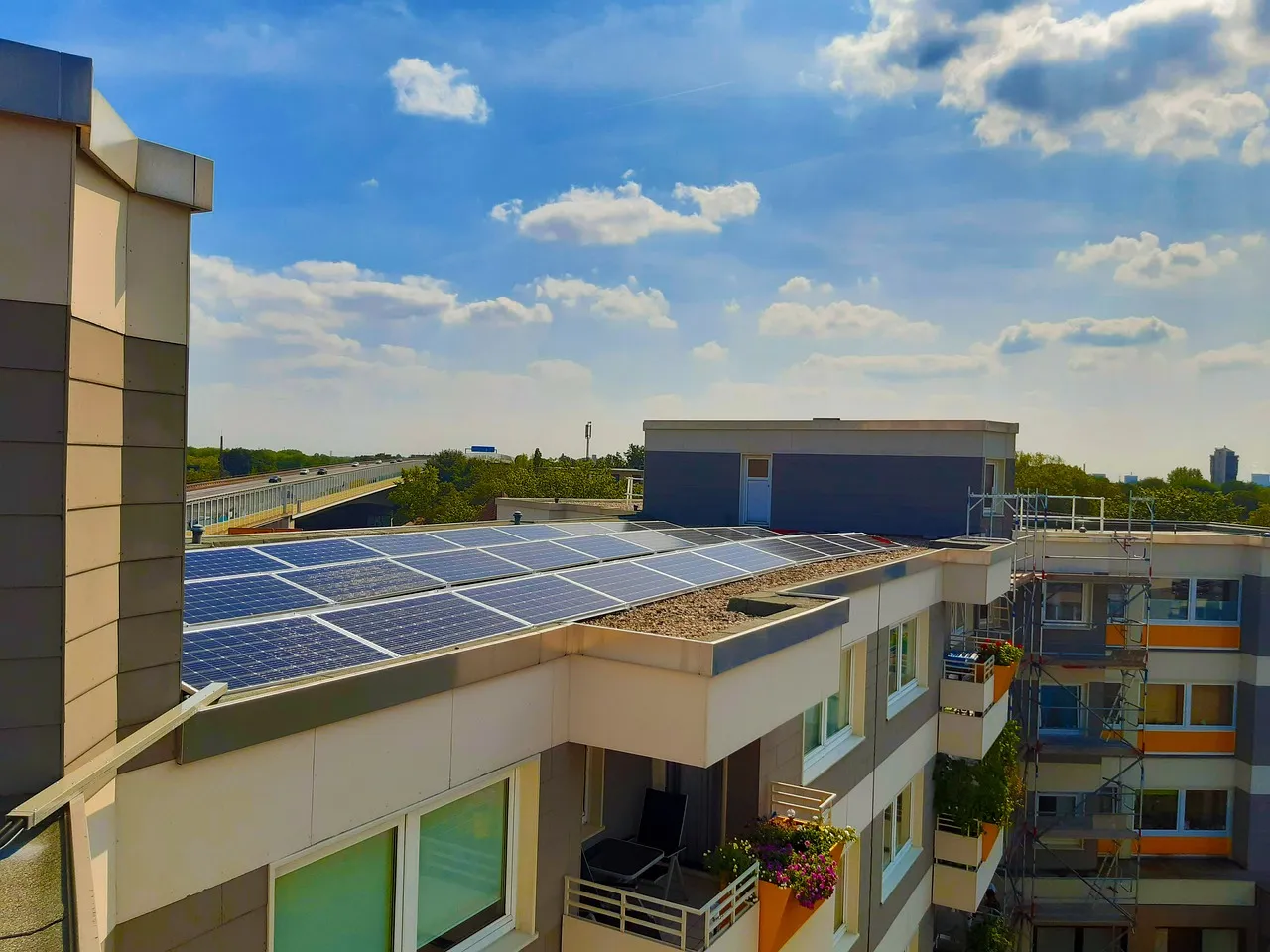
ThinkingEarth’s Role in Transforming Greece’s Urban Energy Landscapes
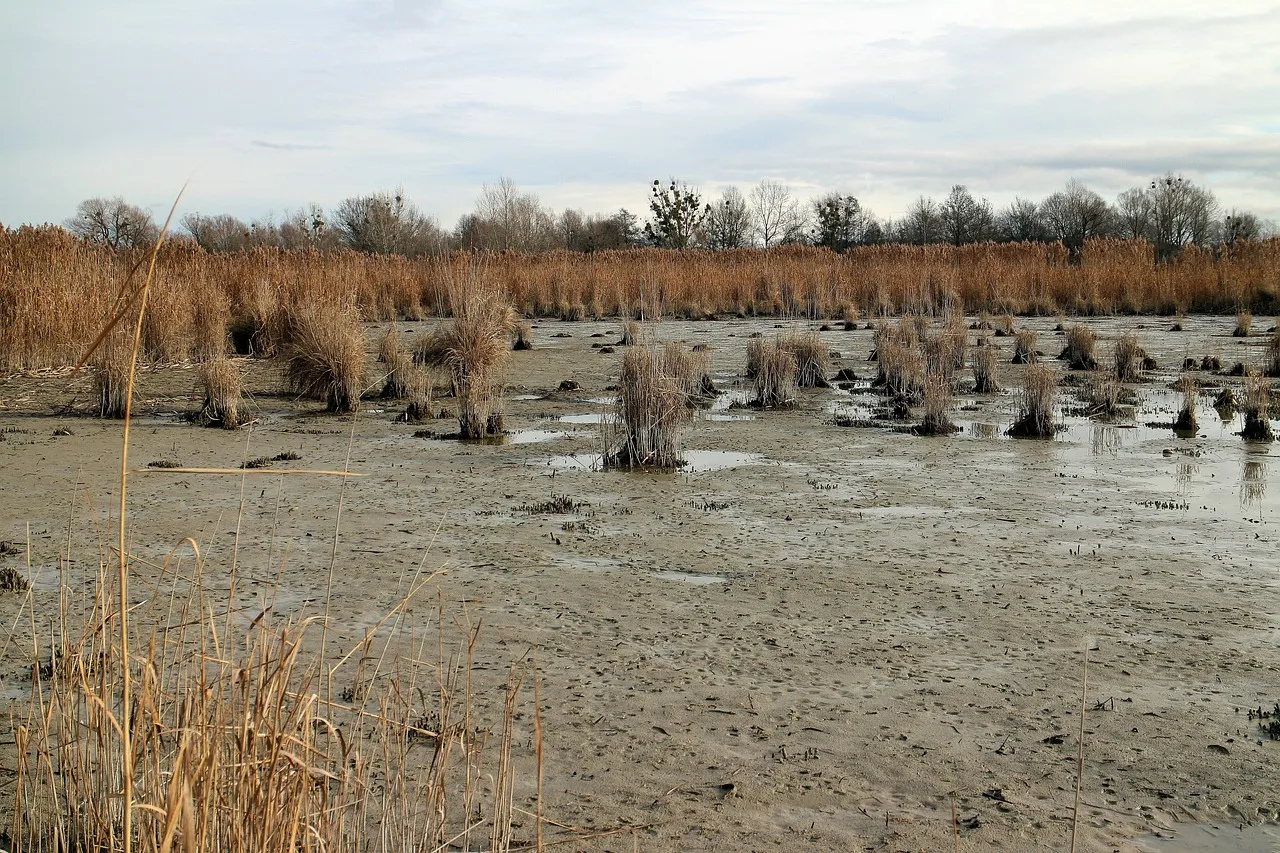