ThinkingEarth: Exploring self-supervised learning techniques
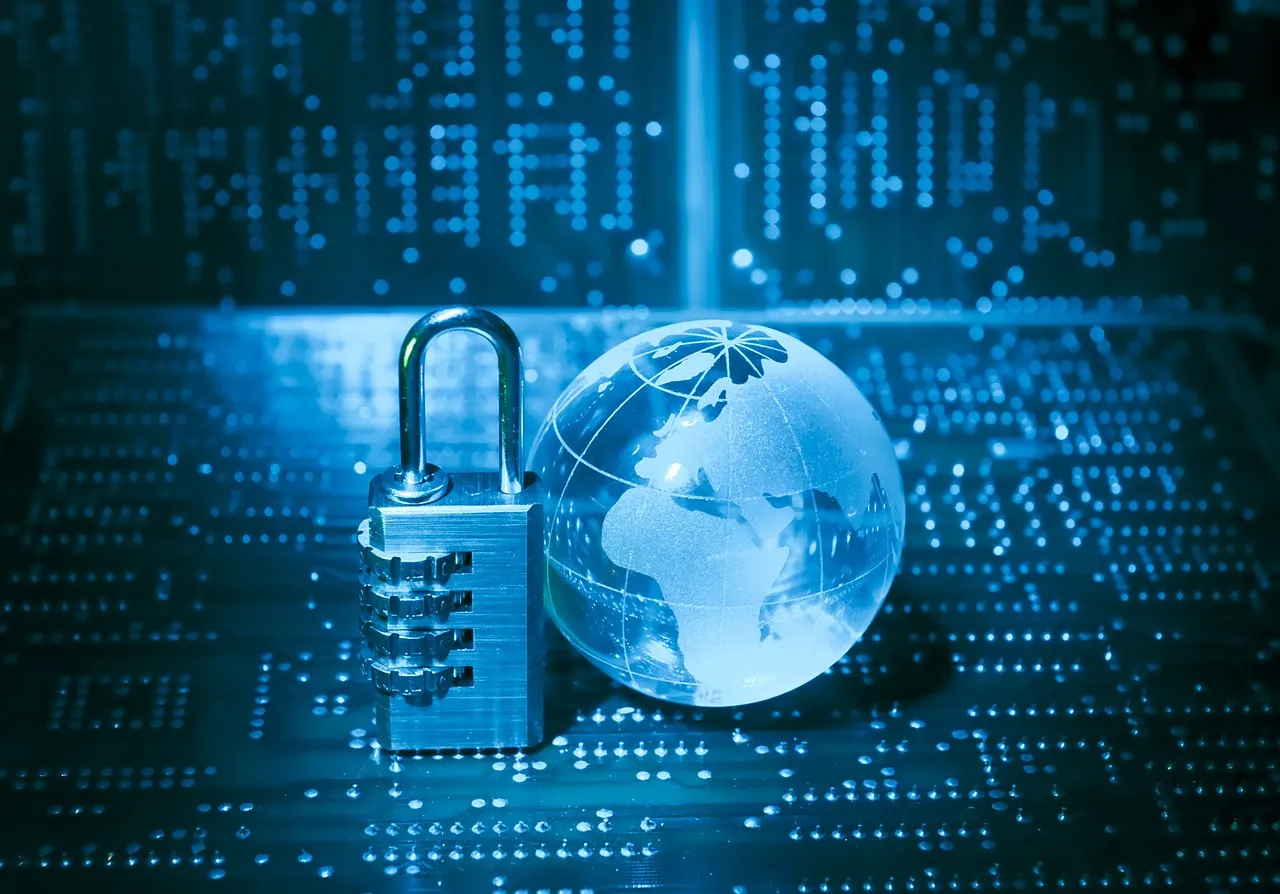
However, valuable resources like global land-use data and powerful vision models are often overlooked. The publication “Multi-label Guided Soft Contrastive Learning for Efficient Earth Observation Pretraining “, co-authored by ThinkingEarth partner Xiao Xiang Zhu, shows that using these free resources can overcome common issues in contrastive learning and improve the efficiency of EO pretraining.
Specifically, they introduce soft contrastive learning, which uses multi-label supervision from land-cover data to better handle multiple positive matches in complex scenes. They also explore cross-domain continual pretraining using multispectral and SAR imagery, leveraging top vision models like DINOv2. By incorporating simple techniques like weight initialisation and Siamese masking, they achieve strong pretraining results, even with different input types and channels.
A lot of research has focused on developing Earth observation (EO) foundation models, mainly by adapting self-supervised pretraining techniques for EO data. Early studies focused on creating contrastive views from EO data.
What is ThinkingEarth doing with self-supervised learning
We’ll focus on improving self-supervised learning for Copernicus Sentinel datasets, especially for contrastive learning and masked image modelling in Sentinel-1, 2, and 3 data compression. Our approach will develop methods to combine self-supervised representations from different Sentinel data types, keeping important information while considering which data type is most dominant.
We also aim to boost the ability of deep learning models to generalise across different locations and times using self-supervised learning, meta-learning, and continual learning techniques.
Share
Read next
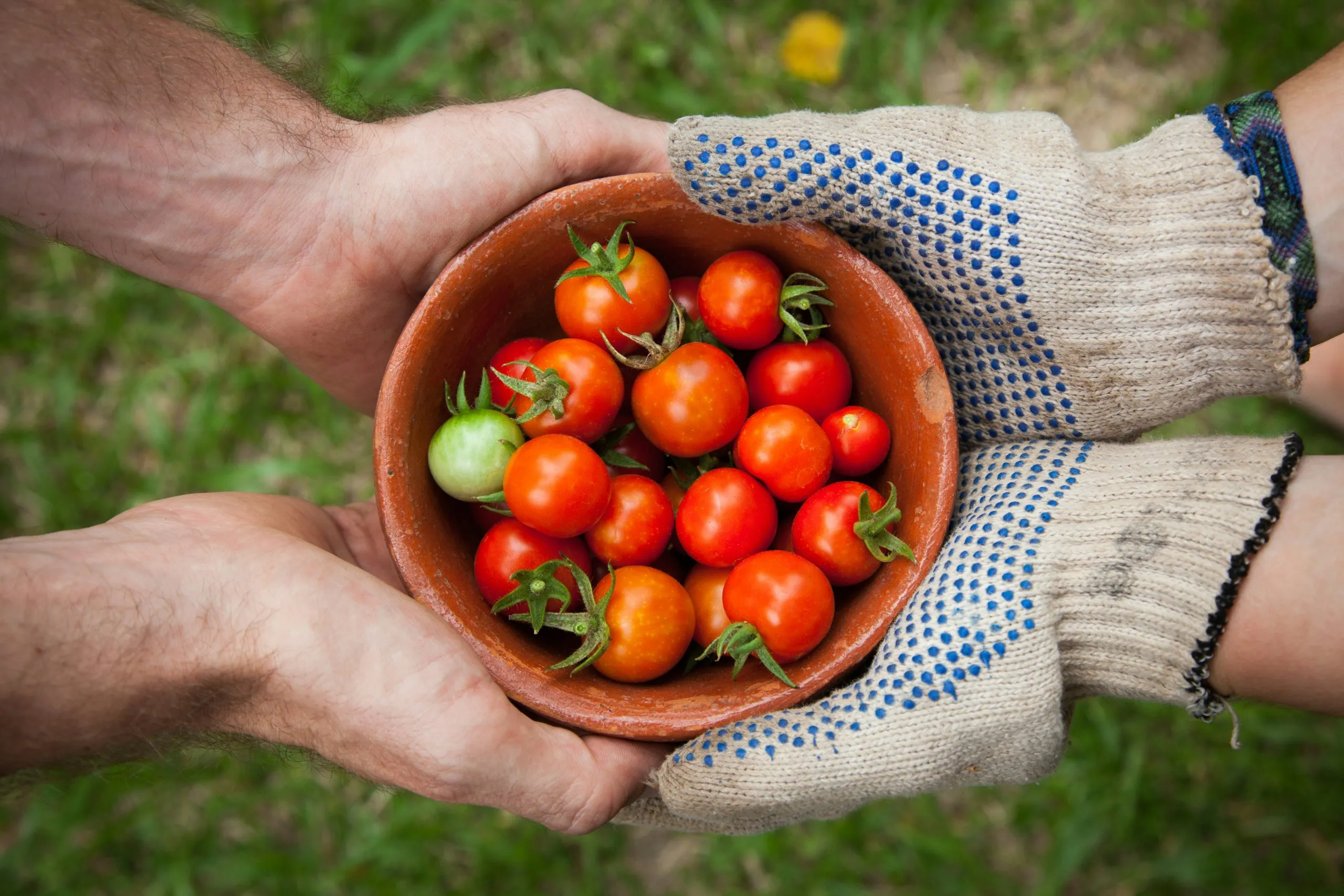
Tackling Food Insecurity with ThinkingEarth
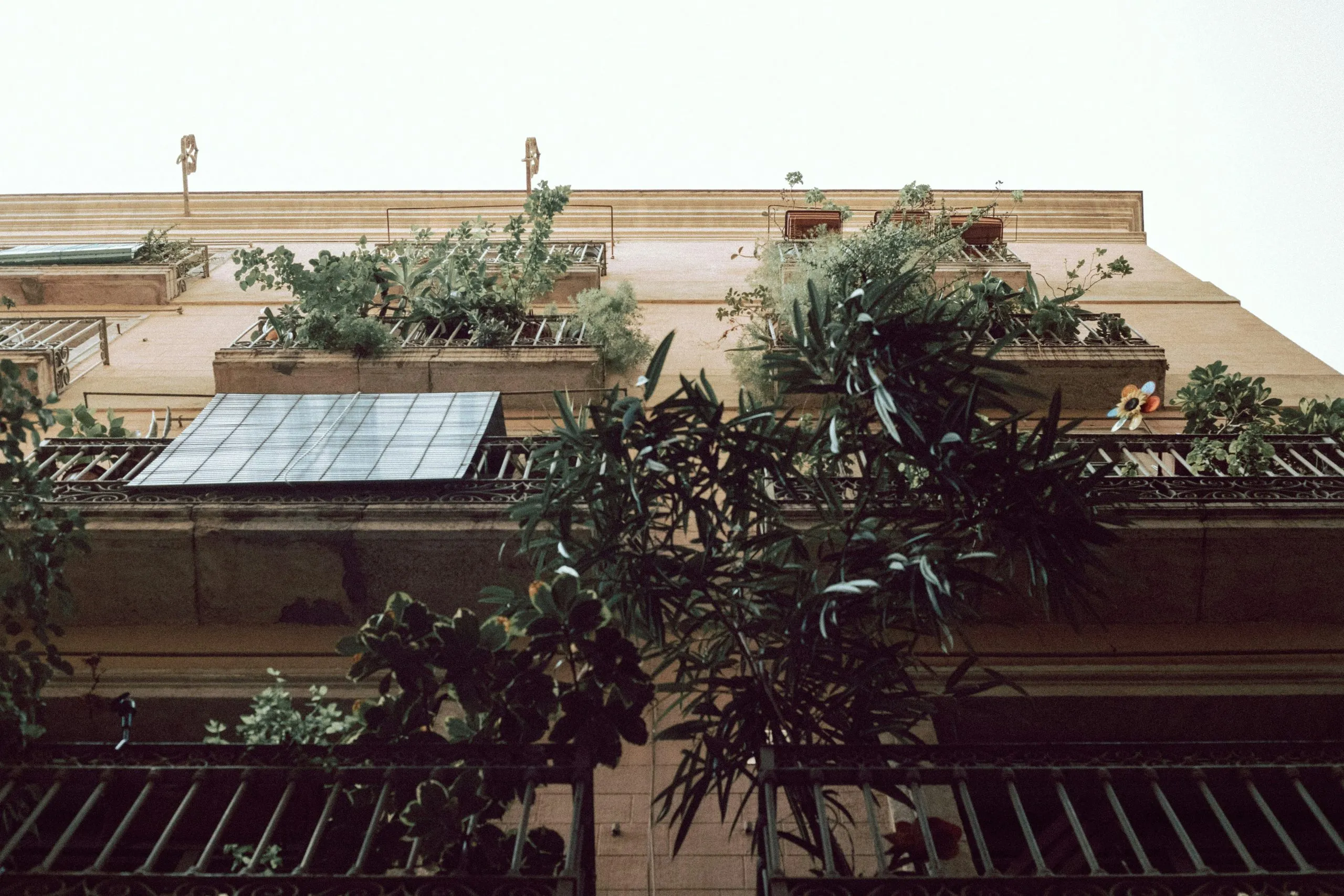
A Smart Grid Future with ThinkingEarth
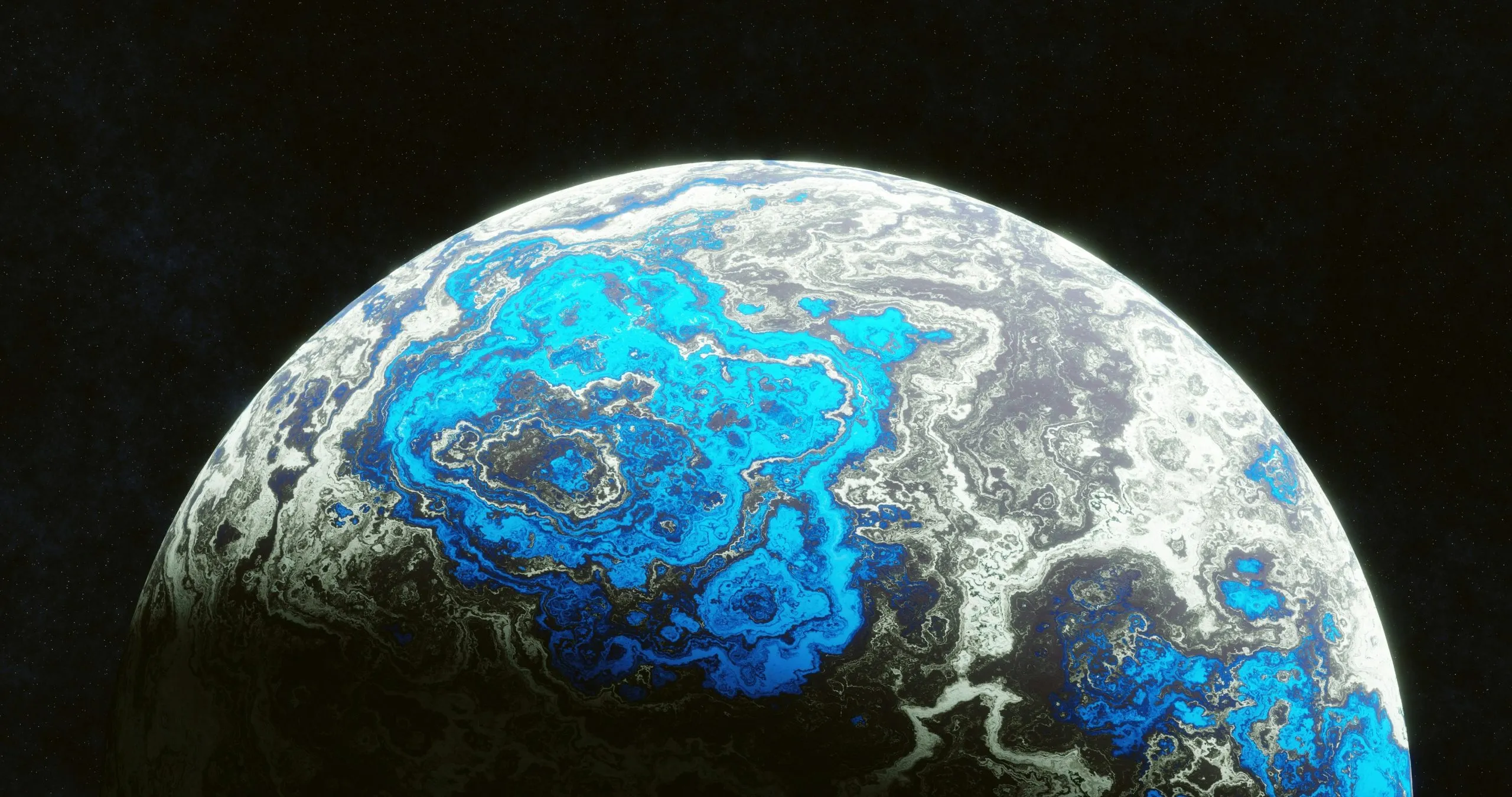